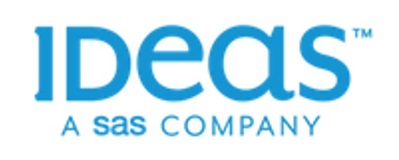
Three things hoteliers need to know about machine learning
By cameron in Uncategorized
When it comes to hospitality analytics, it’s hard to ignore the recent airtime devoted to and levels of interest in the topic of machine learning. The industry has been humming with how machine learning, specifically, helps hoteliers streamline much of their current time-constrained, manual processes – freeing up valuable time to focus on their revenue strategy in the process.
NB This is a viewpoint by Alex Dietz, advisory product manager for IDeaS Revenue Solutions.
And help hotels it does. Today’s analytics are using data mining, machine learning and statistics to deliver the most optimal pricing and inventory decisions for hotels.
One thing, however, is certain: just don’t call the rise of machine learning a comeback. While machine learning has been making waves in recent discussions, its methodologies have actually been used in the field of predictive analytics for many years.
Its more recent revival, on the other hand, is in large part reinforced by breakthroughs in the ability to automatically apply complex mathematical calculations to today’s large volumes of big data.
So what exactly do hoteliers need to understand about machine learning in revenue technology? Here are three things today’s hotels need to know about the role machine learning plays in revenue management analytics:
Machine learning can refer to many different types of applications, in many different industries
Machine learning has many different examples in today’s world and it can be applied to many different types of scenarios. Some of the more popular examples of machine learning throughout various industries include personalized Netflix recommendations, Malware detection programs, and proactive product suggestions from the likes of Amazon.
In revenue technology, machine learning is often used in conjunction with statistical methods to produce cutting-edge forecasting and decision optimization. High-performance technology can use machine-learning processes to better understand the relationship between price and demand, and generate rates that adapt and anticipate market fluctuations.
The combination of such powerful and proprietary analytics gives hotels valuable business insights, intuition and understanding of their data – and the opportunities it presents them with.
Machine learning is just one piece of the analytics puzzle
Within the realm of predictive analytics, machine learning is a method that can be applied to different types of scenarios to quickly evaluate, predict and optimize outcomes. Common scenarios for its application involve those lacking human expertise, or a way to describe or organize the experience, such as facial recognition software.
However, there are also scenarios in which a business problem is not an ideal candidate for machine-learning processes. An example of this is when a theoretical model already exists, and there’s a clear understanding of how certain factors impact the solution.
Consider the models used to forecast demand or optimize revenue for hotels. Revenue managers often ask “why is my forecast so high?” or “why was this rate selected?” The ability to quickly assess and answer these types of questions comes from knowing exactly what data these models use, and how they use that data.
In the case of machine learning, these questions would be difficult to answer and could literally change from one hotel to another. For situations where we need to understand why one resolution is better than another, machine learning is not going to be the ideal approach.
Machine learning – and revenue technology in general – still needs human interaction
Machine learning technology organizes and analyzes data in seconds, and more sophisticated revenue systems automatically deploy business decisions based on this type of analysis. But one of the critical components for hoteliers using these advanced solutions is recognizing that even though predictive analytics streamline and automate processes, there is still a need for human interaction. Hoteliers will still need to validate actions and alert the technology to things it cannot anticipate.
NB1 This is a viewpoint by Alex Dietz, advisory product manager for IDeaS Revenue Solutions. It appears here as part of Tnooz’s sponsored content initiative.
NB2: Image by BigStock